Picture a world where you could recognize medical problems before sickness symptoms appear to your doctor already knowing the illness source. The physician would already have identified the disease based on medical examination. Gene composition determines all treatment options in such a medical environment. The market introduction of life-saving medications would shorten from years to just months. Machine learning provides such capabilities to healthcare applications.
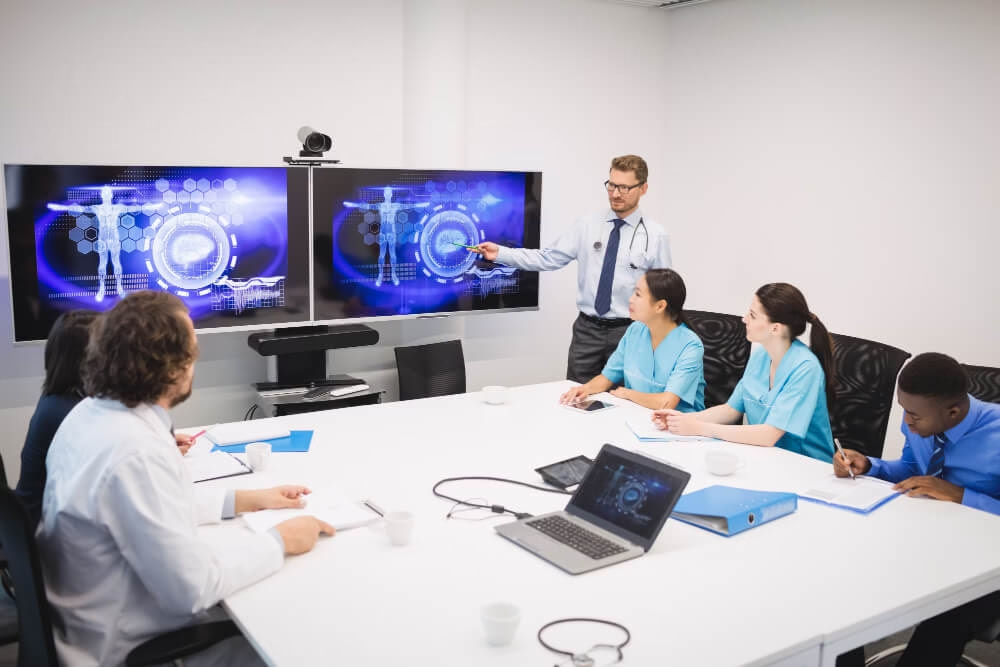
Machine learning uses rapid and precise evaluation of extensive data collections. This, in turn, helps the medical practitioners with better choices. The technology reduces expenses while generating better profits yet proves essential for deciding between patient fatality and patient recovery. Research suggests artificial intelligence will conserve healthcare dollars to the amount of 150 billion annually. The research indicates that this advancement will be possible starting from 2026.
The importance behind this paradigm transition requires explanation. Historically medical images together with patient histories proved challenging to deal with as healthcare data. Modern ML technology makes possible the advancement of earlier healthcare obstacles. Computers learn to recognize patterns. The combination of predictive capabilities and precise outcomes happens faster through machine learning techniques than possible at any earlier time.
Learning about Machine Learning in Healthcare
What Is Machine Learning?
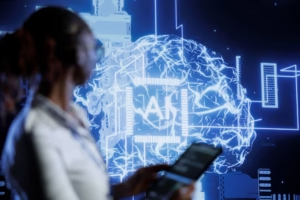
Artificial intelligence features machine learning as its branch, which allows systems to improve their operations through experience without preset instruction. Humans use data to learn patterns that machines can replicate through the teaching process for decision-making in much the same way. ML systems have the ability to continuously learn and evolve with extra information because they do not function through hardcoded programming rules, thus making them ideal for healthcare settings, which need dynamic processing of abundant data.
Why Healthcare?
Medical institutions generate significant amounts of information through patient files and imaging reports together with genetic data and patient responses. The vast amount of healthcare data went unused for many years. However complex and overwhelming the data proved to be for human analysis. Machine learning depicts a system that operates efficiently with extensive data volumes. The conversion of raw data into meaningful information through this process supports health improvements.
Sepsis causes over 11 million annual fatalities because it signifies a life-threatening medical situation. Medical professionals typically fail to detect sepsis early through conventional sepsis detection methods. Patient data processing through ML algorithms enables sepsis prediction before symptom onset during hours before clinical manifestation. Early detection through this system helps medical staff preserve patients’ lives.
How It Works
The main categories of machine learning operations in healthcare include:
- Supervised Learning: All algorithms need training data which requires specific labels. By analyzing many X-rays, an ML model can learn to detect lung cancer. X-rays undergo marking during the process to classify them as “cancerous” or “non-cancerous.”
- Unsupervised Learning: This method identifies concealed data patterns which lack manual classification. Unsupervised ML employs data grouping mechanisms for patients with similar genetic profiles to suggest individual treatment options.
- Reinforcement Learning: The learning process occurs through repeated trials and errors, which enhance operational performance based on provided feedback. Robotic surgical assistants develop enhanced precision because they use acquired feedback to conduct self-learning processes.
Now, let’s look at some of the types of learning and their work in different fields.
Types of Machine Learning in Healthcare
Type of Learning | How It Works | Example |
---|---|---|
Supervised Learning | Learns from labeled datasets. | Diagnosing cancer using annotated medical images. |
Unsupervised Learning | Identifies hidden patterns in unlabeled data. | Clustering patients for personalized treatment plans. |
Reinforcement Learning | Optimizes actions through feedback and rewards. | Training surgical robots for improved precision. |
Machine learning serves both technological advancement and empowerment for healthcare providers as well as patients. ML combines human knowledge with algorithmic intelligence. The healthcare system becomes smarter through this development. The current system demonstrates increased empathy.
Finding Diseases Early: Spotting Illnesses Before They Happen
The Strength of Predictive Analysis
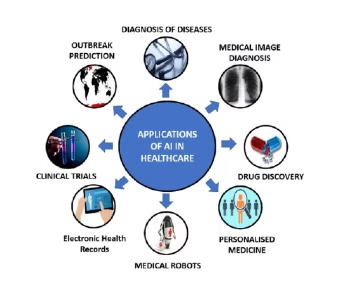
The ability to detect disease risks before experiencing symptoms would be possible through certain knowledge. New information enables you to implement preventive measures. The development of machine learning allows such progress to become achievable. Small disease indicators that may develop in patients can be uncovered by examining their genetic data and behavioral patterns alongside medical records.
Regular mammograms fail to detect breast cancer early signs sometimes because they have limited accuracy. DeepMind, under the ownership of Google, delivers cancer detection at a 94.5% accuracy rate, which surpasses human physicians in early disease identification. The improved rates of survival along with the decreased need for avoidable medical tests are a result of this advancement.
Filling Holes in Diagnosis
The identification of incorrect medical diagnoses signifies a major issue that affects healthcare delivery. The World Health Organization records diagnostic errors as affecting millions of people yearly who later get improper or delayed medical treatments. Medical machines decrease diagnostic errors by offering information-driven secondary consultations.
The healthcare system benefits from IBM Watson Health’s utilization of ML to analyze radiology reports. It generates possible diagnostic results, which minimizes the chances of incorrect medical conclusions. This research showed Watson recognized diabetic retinopathy symptoms in 87% of patients before the condition advanced to the blindness-preventing stage.
Real-World Applications
- Cardiology: ECG data processing with ML algorithms enables the prediction of heart attacks before their symptoms escalate into critical conditions.
- Neurology: The NeuroPace device enables epilepsy patients to gain more autonomy by forecasting incoming seizures.
- Infectious Diseases: Healthcare organizations used the ML system BlueDot to discover disease outbreak areas so they could implement speedier control strategies during the COVID-19 pandemic.
The application of early detection techniques results in life savings besides healthcare cost reductions. Such detection approaches lower medical expenses and lead to significant cost reductions in healthcare systems. Machine learning advances core diagnostic efforts because of its reinforcing value.
Tailored Treatment
Customizing Medicine for People
Each patient shows different responses to medical treatments. Every individual maintains a unique health status because of genetic components, environmental elements, and personal customs. Through machine learning, healthcare professionals develop unique treatment strategies that match each individual patient. The mechanism studies various differences among patients before matching them to optimal therapy options.
Genes decide how medications affect individuals through the analysis known as pharmacogenetics. Through analysis of genetic data, machine learning determines a patient’s reaction possibility to specific medications. This tool helps find both positive and negative drug responses in patients. Foundation Medicine serves as an illustration of how doctors pick cancer treatments by examining tumor genetic information.
Changing How We Manage Long-Term Illnesses
Patients who have diabetes or hypertension need prolonged medical treatment. The medical application MySugr uses ML algorithms to process diabetes patients’ glucose data and behavioral patterns, which results in individualized healthcare advice. Patients gain health control through these systems, which concurrently lighten healthcare system loads.
Real-Time Adaptations
The learning process of machine learning continues beyond its first recommendations through a process of adaptation. The Fitbit and Apple Watch collect actual time-based measurements of heart rate alongside physical activity data from their users. The processing of such data through ML algorithms supplies adaptive feedback by recommending stress management approaches as well as changing fitness goals.
Key Benefits
- Improved Outcomes: Every treatment delivered to patients is tailored to tackle their individual requirements.
- Reduced Side Effects: Precision medicine minimizes adverse reactions.
- Enhanced Patient Engagement: Healthcare services made personalized can create trust between medical staff and their patients which leads to better compliance.
Example
Immunotherapy within cancer treatment enables patients to get effective help against cancer cells from their immune system. The treatment does not yield optimal results for all patients. These learning models estimate which patients will gain benefits from this therapy. The Parker Institute for Cancer Immunotherapy produces these models. The approach allows patients to access effective treatments while preventing them from receiving therapies that will be useless to their condition.
The application of machine learning now concentrates on individual patients rather than conventional approaches. The medical field receives new methods for disease treatment through this technology. Machine learning changes healthcare professionals’ understanding of patients by recognizing their individual needs as well as their personal narratives.
Faster Drug Discovery
Old Ways of Finding New Drugs
Historically, drug discovery has shown itself to be incredibly slow. This process has proven costly by requiring 10–15 years and has required billions of dollars. The process demands multiple experimental tests yet culminates in many failed results. Machine learning applies transformation to the drug discovery process and decreases associated expenses by improving predictive capabilities.
Speeding Up the Search for New Medicines
Drug discovery requires the discovery of compounds that hold potential against specific diseases. The machine learning models created by Atomwise evaluate vast numbers of molecular structures. The models pinpoint molecular compounds that show the highest potential to connect with target proteins effectively.
The early stages of the COVID-19 pandemic saw researchers benefiting from ML tools for their work. The tools enabled quick discovery of possible antiviral compounds during this period. The shorter timeframe compared to conventional procedures made it possible to achieve this task. Through their method they examined potential candidates at an early phase which accelerated their vaccine and therapeutic development.
Lowering Risks in Clinical Trials
Drug development expenses reach their peak point during clinical trials. The analysis of patient data using ML models helps find individuals who will gain most from new drugs, thus enhancing trial success rates.
The ML tools at GNS Healthcare help researchers find particular biomarkers that show how different patient groups will respond to treatment. The level of precision helps remove unsuccessful trials, which leads to the successful market release of highly effective medications.
Real-World Applications
- Cancer Research: Machine learning aids in developing molecules that can stop the development of cancer pathways.
- Rare Diseases: The AI-powered platform Benevolent AI specializes in rare disease research which traditional research avoids because their commercial potential is considered too low.
- Antibiotic Resistance: Through predictions of bacterial resistance development ML models allow antibiotic developers to create the next generation of novel antibiotics.
Drug discovery progresses through the implementation of ML programs. The use of data-driven techniques now leads to more efficient drug discovery processes rather than the prior labor-intensive gambling approaches. Pharmaceutical innovation progresses through a modern era because of this change.
Now, let’s check out a table to more clarify the difference between traditional and ML-driven drug discovery:
Aspect | Traditional Drug Discovery | ML-Driven Drug Discovery |
---|---|---|
Timeline | 10–15 years | 3–7 years |
Cost | $2–3 billion | Up to 70% lower |
Data Processing | Manual and time-intensive | Automated and efficient |
Lead Compound Identification | Relies on trial-and-error methods | Identifies potential compounds quickly using predictive models |
Accuracy | Prone to errors due to human limitations | High accuracy with advanced algorithms |
Scalability | Limited to a few compounds at a time | Can process thousands of compounds simultaneously |
Examples | Traditional labs and wet-lab experiments | BenevolentAI, Atomwise, Insilico Medicine |
Tackling Problems and Ethical Issues
The Good and Bad of Relying on Data
Machine learning thrives on data. The utilization of extensive health-related sensitive information creates significant privacy together with security risks. The dangers of maintaining crucial health-related information became clear after major data breaches in healthcare facilities. Organizations involved in healthcare must meet all the requirements of the Health Insurance Portability and Accountability Act for compliance. Organizations need to follow GDPR (General Data Protection Regulation) for protecting patient data.
Unfairness in Algorithms
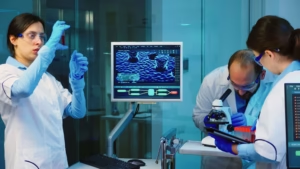
A machine learning algorithm receives its inherent bias from the training data it accesses. Healthcare data that exists today includes historical biases because several segments of the population, especially minority groups, lack representation, leading to unequal results. An ML system designed for hospital patient care prioritization at a U.S. hospital system accidentally preferred white patients to Black patients because its training data contained biases.
Laboratory developers need to use adversarial debasing processes together with regular fairness audits to handle this issue. The collection process must include diverse participants to achieve healthcare results that are fair for everyone.
Dealing with Rules and Regulations
One of the most controlled industry sectors is healthcare because human existence depends on it. The pace of technological progress outpaces the current regulations that govern the healthcare industry. ML-based healthcare tool developers face difficulties when trying to obtain regulatory authorizations from the FDA. The approval process mandates thorough testing and validation for these bodies.
Ethical Problems with Automation
The implementation of ML-powered automation brings better operational efficiency and job elimination risks, especially in healthcare administrative positions. The implementation of ML automation needs to balance human supervision for both building trust among healthcare stakeholders and ensuring ethical practice standards.
Gaining Trust by Being Open
Patients and healthcare providers must trust ML systems because they need full transparency in their operating processes. The process of XAI (explainable AI) helps make complex algorithm decisions transparent so people better understand the systems and improve their acceptance. Diagnostic tools that use XAI systems show to doctors precisely what factors triggered their condition identification. The technology functions as a helpful tool rather than an unexplained device.
Proactive solutions implemented by the healthcare industry will lead to responsible machine learning implementation. The implementation of these systems at their full capacity will lead to utmost life-saving potential. This approach will generate both beneficial and unwanted side effects.
AI Innovations in Healthcare
Predicting Health Needs
Medical care will progress from illness treatment into disease prediction along with prevention. Medical algorithms evaluate patient information to predict upcoming health dangers for doctors to offer timely interventions.
The predictive model developed by Google’s DeepMind Health demonstrates successful detection of acute kidney injuries through its current operations. These tools that offer doctor alerts spanning 48 hours beforehand help to save patients and reduce healthcare expenses.
Advanced ML models equipped in wearable devices will become crucial components for modern healthcare systems over the upcoming years. The combination of Fitbit and Apple Watch devices provides heart attack and stroke prediction through persistent vital measurement tracking and data pattern analysis. The combination of early warning systems improves patient health management abilities, thereby alleviating stress on healthcare facilities.
New Levels of Personalized Medicine
The current utilization of personalized treatment can deepen through upcoming developments in ML. ML algorithms will develop hyper-specific treatments using patient health records and environmental factors together with genomic data. Contemporary cancer therapy approaches will direct their action beyond merely addressing disease type. The treatment plans will analyze specific genetic compositions found in individual tumors. This will improve effectiveness.
The AI platform NVIDIA’s Clara Genomics speeds up genomic sequencing along with analysis to create future medical breakthroughs. The advancement of this technology will lead to personalized medicine becoming a widespread clinical practice, thus eliminating the use of generic treatment approaches.
Using Blockchain with Machine Learning
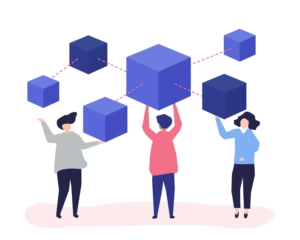
A sophisticated data protection solution emerges from integrating blockchain and ML technology in healthcare. Blockchain maintains clear and permanent health records through its system along with ML software that extracts valuable insights from these records. The partnership between blockchain and ML systems will create trust along with improved data sharing that combines security measures for innovative solutions and privacy protection.
Monitoring and Supporting Mental Health
The conversational AI systems of Wysa and Woebot are now available through ML-powered mobile applications that offer mental health assistance. Next-generation NLP technologies will be added to future developments. The platform can detect early symptoms of both depression and anxiety as well as stress symptoms. SLCs and behavioral indicators will reveal the detection of mental health conditions.
Your smart assistant will recognize behavioral and speech patterns in the future world. The application provides up-to-date mental health resources to users. This connection platform helps users reach professionals. These instruments show potential to transform the way humanity presents itself to mental well-being intervention.
Conclusion
Healthcare now experiences a fundamental paradigm shift because machine learning entered the healthcare arena through technology integration. Through such applications of ML technology, doctors can recognize diseases at their earliest stages. It utilizes personalization features for treatment creation and speeds up the process of discovering new drugs. Modern technology, through machine learning, extends new boundaries in medical practice while at the same time protecting lives.
However, as with any transformative technology, its success hinges on responsible implementation. The delivery of equal healthcare for everyone depends on addressing ethical matters and maintaining both transparent machine systems and inclusive data usage.
Prospects for innovation look bright because many exciting ends continue to appear. Businesses should support all AI healthcare innovations that serve technological excellence and human well-being when we move ahead with AI excellence.
References
- “Transforming Patient Outcomes: Cutting-Edge Applications of AI and ML in Predictive Healthcare” – SSRN
- “Distributed Technologies Using AI/ML Techniques for Healthcare Applications” – ResearchGate
- “AI and ML Digital Transformation on Cardio Applications in Healthcare for Outpatients’ Safety” – ResearchGate
- World Health Organization
Reports on global healthcare advancements and ML applications in improving patient care. - National Institutes of Health
Insights into ML contributions to precision medicine and clinical trials. - Statista
Statistical data on the growth of AI and ML in healthcare industries. - “The Ethical Implications of AI in Healthcare” – Journal of Medical Ethics
Articles on balancing innovation with patient trust and data privacy.