The growing concern for privacy stems from data breaches and acts of surveillance across contemporary society. According to the reports we often hear, Our personal information remains at risk whenever we use the internet. Could we achieve both personal data protection and artificial intelligence capabilities at the same time? The concept behind federated learning makes it possible. Federated Learning is a new approach to enabling machine learning through protected data storage on individual devices.
So, what is federated learning? Federated Learning technology makes it possible to maintain exclusive data storage rights within your electronic devices. First-party devices maintain their own personal data within local storage. With federated learning, your device maintains sole access to personal information regardless of what data it contains. With decentralized machine learning, each device processes its data autonomously without sharing its insights with central servers. Each device achieves increased intelligence and performance through this approach. Devices upload better performance through central servers. Your data remains secure, while controlled access decreases the risk of purposeful misuse at the same time.
How Does Federated Learning Work?
Suppose a classroom that contains students who each handle one unified project while maintaining personal note secrecy. Each student explains their important points to the teacher when the assignment is complete. The teacher applies these reported main points to improve the project. Each device handles personal data independently and directs the model updates through secure server transmission for central analysis in federated learning. The process enables the upgrade of shared models that produce better results for everyone while maintaining user privacy.
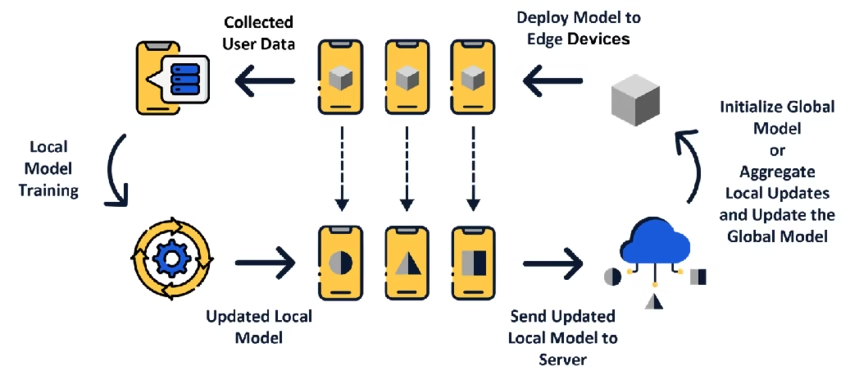
Your data remains stored on your device through distributed learning because this method differs from standard machine learning approaches. The updates travel to the central server using differential privacy resources, which secure the user’s identity from being exposed even during third-party access attempts.
Apple’s Use of Federated Learning
The predictive text features on the iPhone get better with Apple implementing Federated Learning. The way this algorithm operates demonstrates one of Decentralized Machine Learning’s practical applications. Type functions on your device allow learning to occur while data stays private from Apple servers. The system sends back updates to Apple so the company can enhance its AI without gaining access to user data.
How Federated Learning Keeps Your Privacy Safe
Data collection has become ubiquitous in current society, making privacy as critical as ever today. We know the risks: Data breaches, together with spying and company activities that sell user information, represent major privacy threats. People confront the biggest challenge of using smart technology without compromising personal privacy.
Federated Learning is a solution. The technology enables artificial intelligence systems to learn independently of collecting data in a single centralized space. AI functions through federated learning as a method that prioritizes privacy for all users.
How Data Remains Private
According to federated learning, your data remains permanently within your device. Your own laptop or smartphone examines data directly from your device. Your device analyzes your behavior as you use apps and type keyboard inputs directly on your machine instead of transmitting these data points. The central server receives the AI improvements as the sole output from device-level operations. With this approach, your personal data remains protected because it does not reach any other party.
No Personal Data Shared
The primary advantage of using federated learning is you do not need to hand over your personal data. Regular machine learning exposes your data as it flows from your device to central servers, which creates security risks. Through collaborative learning systems, extract knowledge from your data while keeping your data protected. Your phone’s voice assistant uses your voice without sharing it with the company when you speak to it. Although personal information remains protected, the assistant continues learning. By using this technology, you gain better artificial intelligence without exposing your private information.
Reducing Privacy Risks in a Digital World
The quantity of our digital service usage exposes our personal data to increasing risks. Using digital services results in creating precious data, which could face potential misuse with each interaction or internet search. Through federated learning, organizations maintain full control of their private databases. Your data remains secure through strong encryption together with methods that protect privacy.
Statistical research highlight the magnitude of data privacy problems. Gamma Analytics predicts global cybercrime will cost the planet over $10.5 trillion annually before 2025. The need for privacy-enabled solutions such as Federated Learning arises because it guarantees privacy protection while augmenting powerful AI system development.
Through federated learning, users gain access to AI functionality while maintaining complete privacy protection. The approach represents an exciting technique for resolving current privacy problems. The technological advancements will foster better protection for our digital personal information into the future.
The Benefits of Federated Learning: Good for Data Privacy and AI Growth
Federated Learning represents an innovative approach to protecting our information safely. Through federated learning we can maintain the privacy of personal data without compromising our ability to utilize AI effectively. Privacy becomes particularly essential in fields such as finance and healthcare because individuals depend on it strongly. Additional advantages from federated learning support both AI functionality and create better trust between users.
Better Data Privacy and Security
A primary advantage of federated learning consists of its capability to preserve user privacy. Users benefit from direct device data storage since storage on central servers leads to security vulnerabilities. Data privacy is significantly enhanced because valuable personal information remains on user devices.
Under federated learning, healthcare providers can train medical artificial intelligence systems while maintaining strict confidentiality of patient information. Healthcare institutions use their native patient records to enhance medical predictions and therapies through secure patient information protection approaches.
Better Personalization
Through federated learning, individuals can access customizable user experiences that preserve their privacy. Federated learning operates directly with user device data in order to extract knowledge from individual behavior patterns and preferences. The capabilities of your smartphone’s virtual assistant automatically improve through increasing usage. Your assistants become better at understanding you along with providing better suggestions as time passes.
Using federated learning technology, fitness applications analyze your device-based workout data to generate targeted exercise recommendations. With this approach, the application becomes better suited to reach your individual fitness objectives.
Quicker Updates and More Efficiency
The processing speed increases through federated learning because data remains on individual devices. A central server remains the only destination for traditional data sending because updating requires overall server speeds. The updates are processed directly on devices before sending refined methods to the main server in federated learning systems. Faster development speeds for artificial intelligence combine with decreased data transfer needs.
The real-time driving skills of self-driving cars receive improvements through federated learning processes. Individual vehicles gather information about nearby road conditions directly into their driving algorithm. Cars communicate their updated information to the server, which analyzes and empowers other vehicles to learn simultaneously.
Cost Savings and Scalability
Federated Learning is also cost-effective. The use of central servers for massive data storage generates expensive operational costs. Data localization lowers both storage expenses and processing costs. The system uses resources to update small model sections instead of working with all available data.
An increase in connected devices benefits distributed AI systems directly because of their seamless scalability. The model can undergo improvements through new devices, even when there is no need for centralized data storage. Federated learning proves beneficial for businesses operating at high growth rates, such as fintech and e-commerce.
“The future of data privacy lies in technology that values individual control and collective intelligence—Federated Learning embodies this vision.”— Dr. Jane Doe, AI Privacy Expert
Challenges and Limitations of Federated Learning: What Needs Attention
The potential of federated learning to enhance data security and machine learning applications faces multiple implementation challenges. For comprehensive performance, federated learning faces remaining challenges despite its privacy improvements.
Data Differences and Model Accuracy
The data across devices that participate in federated learning shows substantial diversity. The variations between devices produce visible impacts throughout data quality, together with data volume and usefulness. Some users in a fitness app enter comprehensive workout records, yet other users enter simple workout data. The inconsistent nature of updates created through this variation poses risks to the overall precision of the model.
Federated learning functions optimally when trained with extensive dataset information. The lack of sufficient device data or power resources prevents models from acquiring proper structural knowledge. Resolving these issues demands improved algorithms together with precise techniques for uniting device updates to enhance model performance.
Limited Device Power
Responding devices encounter processing power limitations that prevent proper performance. Modern devices maintain strong performance capabilities; however, their ability to train advanced machine learning models faces possible processing limitations. A model designed to recognize faces presents too much processing challenge for older smartphones or low-power devices to complete training successfully. The analysis of health data becomes a computational hurdle for specific devices.
The distributed AI framework relies on such devices to execute major computational practices. When devices lack resources, the process of learning can slow down as updates become delayed. Developers need to test federated learning performance on scarce resource systems to stop systems from slowing down.
Communication Delays and Network Issues
The system design of federated learning requires multiple devices to transmit their algorithm updates to a central server. Updates sent between devices and the server through a network may experience complications, thereby suspending or reducing system performance. Good performance from the system requires regular update transmissions from numerous devices. Training delays will increase when devices connect to slow network speed zones or intermittent networks.
The length of time information takes to transmit carries major importance in software systems that are extensive in scale. Modern applications that implement smart cities infrastructure or industrial Internet of Things systems demonstrate this restriction at work. Large environments surrounded by excessive data along with updates become challenging to manage. Novel methods are developed to enhance data synchronization as well as accelerate model update processes.
Privacy Risks and Security Issues
Federated Learning introduces privacy advantages yet produces several privacy vulnerabilities. Model update manipulations happen during attacks carried out by untrustworthy actors. The system faces risks because unauthorized individuals make attempts to extract confidential information or to feed it with incorrect data. False updates generated through hacked devices have the potential to damage the performance of a system.
Emergency responder selection cannot be effective without implementing robust protective measures at every stage of distributed AI systems. Scientists study two security methods called secure multi-party computation and differential privacy. The techniques address security as their main objective in federated learning systems. The expanding nature of this technology will unavoidably bring about fresh security threats.
Future Directions: The Role of Federated Learning in Data Privacy and AI
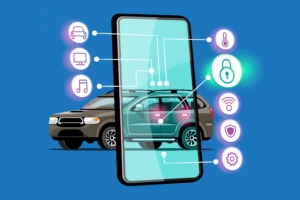
Federated learning will gain greater significance in the future because of evolving technologies, together with AI development requirements and data privacy considerations. Research teams work actively to enhance federated learning functionalities while attempting to resolve its current hurdles. Several promising applications arise from federated learning.
Better Privacy Techniques
Improved privacy technologies are predicted to develop in future years. Federated Learning currently implements differential privacy methods for its functionalities. These privacy methods successfully protect single-user information. The current system needs additional development. New secure processing approaches such as SMPC and homomorphic encryption provide increased protection to data in processing stages. The processing system allows analysts to keep information hidden even when performing calculations.
The processing of encrypted data becomes possible through homomorphic encryption technology. Security keys prevent anyone from extracting data content while carrying information through interception attempts. By combining these methods with privacy-preserving machine learning techniques, they become more powerful for enhancing data security. Sensitive fields, just like healthcare and finance, require particular attention to security because of their nature.
Combining Federated Learning and Edge AI
Federated Learning and Edge AI have formed an important new intersection. Mobile devices and automobiles execute Edge AI by performing computations inside their hardware storage blocks, rather than depending on cloud-based servers. The integration of these technologies permits multiple devices to participate in model training initiatives without compromising either security or offline functionality.
Both technologies enable immediate AI model updates when operators require quick response decisions. Self-driving vehicles use immediate decision-making capabilities. Beyond local storage applications, distributed learning enables these models to incorporate road and traffic data for improvements. Autonomous vehicles maintain operational autonomy alongside data-aware enhancement from shared learning experiences that protect individual privacy information.
Federated Learning for the Internet of Things (IoT)
Further expansion of IoT requires to be federated learning to manage and secure the rising amount of data. The data originates from many connected devices. Smart home systems leverage federated learning techniques to improve functionality without requiring users to transmit their sensitive data to central storage servers.
Smart thermostats utilize user behavior analytics, including temperature modifications together with selecting preferred temperature adjustments to enhance their performance. Under Edge Learning, these devices execute better predictive computations without transferring user data off their device. The model maintains improved versions while preventing the need for centralized storage by distributing new data only between participants, thus ensuring greater privacy protection.
Federated Learning in Personalized Healthcare
The implementation of federated learning creates major enhancements for individual health care programs. Through the implementation of decentralized learning, healthcare providers gain the ability to design patient-specific models that uphold healthcare data privacy.
With federated learning, healthcare providers achieve better accuracy in their personalized cancer treatment models. The models evaluate treatment possibilities that are fashioned specifically to match each patient’s unique medical profile along with their daily activities. Patients retain full privacy protection because their healthcare data remains stored within their local hospital system. Using this system, scientists achieve treatment discoveries by protecting patient information.
Conclusion
Through federated learning, we have transformed our perspectives on data security models and artificial intelligence deployment strategies. Devices can process data independently. Each device transmits only data updates while keeping all personal information secure. Through this approach, artificial intelligence methods learn new information and develop better capabilities.
Using this approach enhances privacy within fundamental domains comprising healthcare together with finance. The distributed processing improves both autonomous vehicle decision-making capabilities along with response times in various smart products. Decentralized machine learning presents a powerful solution that allows individuals and businesses to use artificial intelligence while safeguarding their personal information.
Privacy-Preserving Technologies demonstrate the promising opportunities within its forecasted growth. Multiple upcoming enhancements to privacy technology indicate its promising growth potential. The network will serve as a technical connection between AI functions and devices while adding personalized healthcare benefits. The adoption of this technique by additional sectors will produce novel analytics solutions that unite artificial intelligence features with privacy assurance capabilities. By using this approach, we acquire smarter safeguards for the future.
Scientists will persist in their investigation of federated learning to improve its current technical limitations. Businesses pursuing innovation and privacy protection will find this evolving tool even more useful. The system ensures absolute security of your information. Your data stays protected when you use fitness apps in combination with smartphone assistant capabilities and AI healthcare technologies.
Implementing this contemporary method delivers privacy advancement beyond its core function. We establish a future where artificial intelligence systems team up for privacy-protecting endeavors. The options are endless. Data-local machine learning approaches will undoubtedly become the fundamental standard for data utilization across diverse scientific disciplines.