Global use of artificial intelligence technology continues to expand throughout the world. Scientists have replaced theoretical quantum neural networks with practical implementations. Traditional AI systems demonstrate success, although they cannot efficiently solve complex problems. Quantum neural networks (QNNs) show the novel development achieved by combining quantum computing technology with neural network features. Quantum computing brings the power to revolutionize computing operations and produce quicker outcomes for complex computational issues in various fields.
QNNs are very important. The mechanics of quantum mechanics enable better information processing through QNNs, thus transforming the operative capabilities of normal AI systems. QNNs bring value to many domains because they accelerate drug research and enhance information protection capabilities. The following research investigates QNN operations together with their advantages and special opportunities in detail.
What Are Quantum Neural Networks?
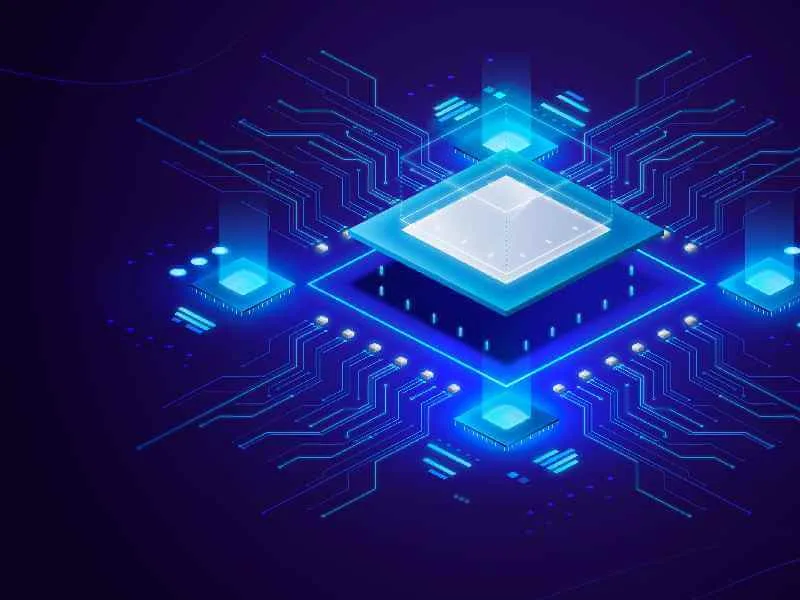
The field of AI consists of a fresh model based on quantum neural networks (QNNs). The model applies quantum mechanics principles through regular neural network architecture. QNNs operate through qubit processing elements rather than conventional computer bits (0 and 1) standard bits. Qubits exist in simultaneous multiple states because of the superposition effect. Quantum neural networks operate simultaneously on multiple calculations because of their ability to handle such operations.
QNNs require the understanding of the key principle of entanglement. The connection of entangled qubits allows an immediate modification of one particle to affect its entangled counterpart, even when these qubits are located at different positions. QNNs utilize this connection to manage information processing efficiently since conventional computing systems cannot do so.
Classical Neural Networks vs. Quantum Neural Networks
Feature | Quantum Neural Networks | Classical Neural Networks |
---|---|---|
Basic Unit | Qubit | Bit |
Neuron Function | Quantum gate manipulation | Threshold-based activation |
Processing | Parallel (via superposition and entanglement) | Sequential |
The process of maze-solving serves as an illustration. When complex mazes appear before neural networks, which analyze paths individually in sequential order, they require extensive time to solve the paths. The QNN system uses simultaneous path analysis for optimizing its solution speed.
Standard neural networks receive advancement through QNN technology because this breakthrough enables superior computational powers. Quantum mechanics empowers their computation to reach results that classical systems cannot attain.
Role of QNNs in Complex Problem Solving
Quantum neural networks (QNNs) are best tool to tackle problems that are troublesome for classical computing systems. They can not replicate the parallel capability of processing enormous amounts of data. They manage very sophisticated calculations with surprising efficiency. They are proving to be very useful solution of some of the most intricate problems faced by industries nowadays.
Optimization
Logistics and supply chain problems have so many considerations in getting the optimal solution. Traditional systems apply stepwise methods that are increasingly difficult to understand as problems become more complex. Traditionally, QNN is different from such systems in that a QNN is empowered to handle many tasks at once, thereby significantly reducing computation time. Planning delivery routes for international shipping, for example, can save dollars, time, and emissions.
Cryptography
Quantum computing introduces both protection issues and modern encryption solutions to the field of cryptography. QNNs play an important role in creating encryption methods that can resist quantum attacks for classical cryptographic algorithms, which show potential vulnerabilities to such attacks. Secure communication channels will be possible after the adoption of quantum computing through these methods. QNNs facilitate instant encryption processes in addition to fast data decryption, which strengthens digital system security.
Drug Discovery
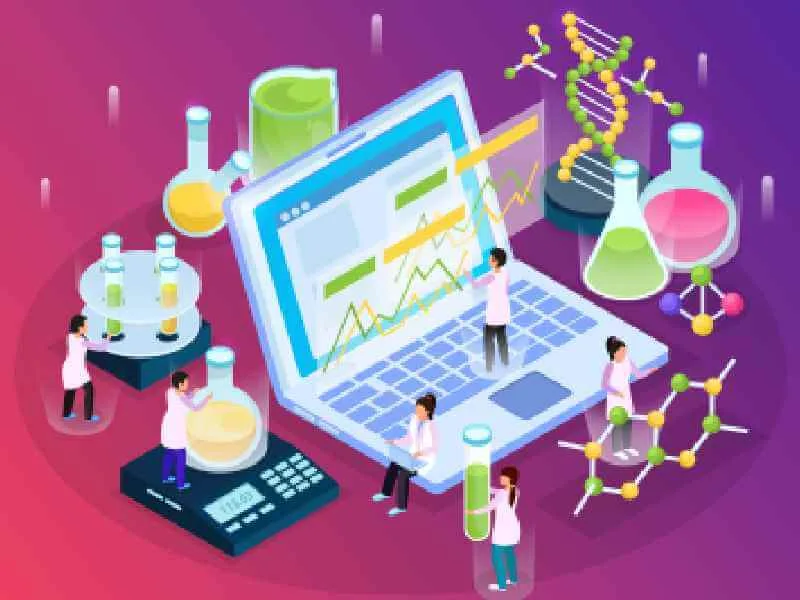
Nonetheless, the pharmaceutical industry has a computer model to design effective medicines. Conventional methodologies fail to simulate more complex molecular interactions because the computers do not have enough power. Quantum neural networks (QNNs) are superior to any computers on this point. They are the fastest in their way of simulating molecular structures and properties accurately. For example, QNNs can find several possible drug candidates for use in just a few days.
QNNs further increase their efficiencies and deliver answers previously thought impossible, thus pushing such parameters further out for what can be achieved with AI in the real.
Advantages of Quantum Neural Networks
Quantum neural networks (QNNs) possess several advantages over classical neural networks. Essentially, they are a uniquely transformative form of computation and artificial intelligence.
1. Unmatched Speed
The computational power of QNNs exceeds the capabilities of classical neural networks because of their advanced processing speed. QNNs carry out parallel operations to process massive data collections and complex mathematics solutions with exceptional speed. Financial modeling highly benefits from real-time analysis, which the implementation of QNNs enables.
2. Improved Efficiency
The basic nature of QNNs makes them efficient because they eliminate redundant processing operations. The holistic approach of quantum number processing differs from sequential task completion methods by using quantum algorithms. Better resource usage combined with shorter problem-solving duration becomes possible because of this efficiency.
3. Scalability for Big Data
As systems grow large with datasets, the classical system always runs into some performance bottlenecks. QNNs, through their parallelism and using exponential computational power, will scale up to power through huge data loads effortlessly. For example, QNNs could make the analysis of global weather patterns or genomics now feasible and efficient.
4. Reduced Energy Consumption
Large-scale problems require traditional AI models to consume surplus amounts of energy. QNNs operate with decreased power requirements owing to their quantum technology apparatus. The reduced energy consumption favors sustainability goals worldwide, which makes QNNs an ideal option for future artificial intelligence development.
The combination of QNN characteristics delivers better operational results to develop sustainable AI applications. The capability of QNNs to revolutionize industries together with environmental protection stands essential for research scientists and developers.
Challenges and Limitations
Quantum neural networks stand to contribute to a truly revolutionized artificial intelligence. That being said, significant challenges do await. These challenges must be solved for mass adoption.
Current Obstacles in QNN Development
- Hardware Limitations: Highly lightweight and unrestrained, quantum computers operate through qubits that are prone to any environmental disturbances such as temperature and electromagnetic interference. The making of such a stable quantum processor is indeed a Herculean task.
- Error Rates: Qubits experience errors because of both quantum decoherence combined with the presence of noise in the system. The correction of such errors needs both advanced algorithms and robust error-correcting codes.
- Resource Intensity: The expenses needed for developing and maintaining quantum systems reach significant financial heights. Significant technical capabilities in addition to substantial financial backing are requirements. Smaller organizations as well as startup businesses find quantum systems unattainable because of their major resource requirements.
Technical and Resource-Based Challenges
- Scalability: When dealing with advanced quantum systems, management of qubits becomes more challenging, and coherence control becomes more difficult.
- Algorithm Development: Early quantum algorithms designed for Quantum Neural Networks need extensive research and development, as well as specialized knowledge for implementation.
- Integration with Classical Systems: Several obstacles exist for connecting classical systems with quantum computing infrastructure. Hybrid architecture optimization proves to be an exceptionally difficult task.
Potential Solutions on the Horizon
Efforts to overcome these challenges include:
- Advances in Quantum Hardware: The development of robust quantum processors involves major technology companies such as IBM and Google. The organizations employ cryogenic cooling methods and innovative qubit design elements for their work.
- Enhanced Error Correction: Scientists work to improve error-correcting codes for reducing discrepancy and noise effects.
- Collaborative Efforts: Quantum research and development processes became more achievable through collaborative efforts between governments, academia, and business organizations.
Future Prospects of QNNs in AI
QNNs demonstrate great potential to redirect the developments of AI applications for the future. Quantum technologies advancing in development will transform various business sectors through their implementation with AI applications.
Predictions for AI Advancements with QNNs
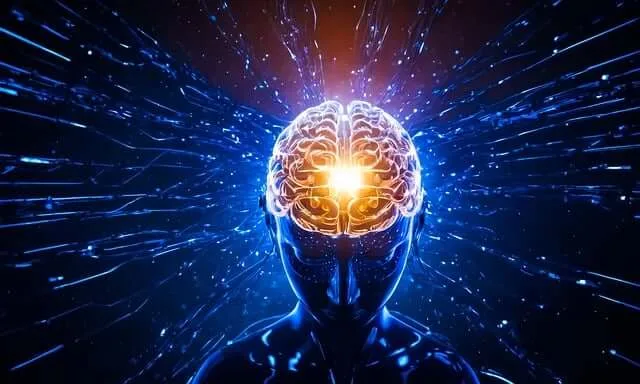
- Exponential Growth in Computational Power: QNN enables the creation of AI models that solve problems previously considered impossible for attainment. These include simulating entire ecosystems. The system will predict challenging global occurrences in addition to simulating entire ecosystems.
- Latest Machine Learning Models: The fundamental design of autonomous systems will improve because of this advancement. Robotics will receive additional growth because of these developments. The pattern recognition capabilities of quantum-enhanced learning algorithms become simpler to perform due to decreased overhead requirements.
Industries Most Likely to Benefit
- Healthcare: QNNs will revolutionize drug discovery through their power to speed up the process of discovering accurate potential treatments.
- Finance: Improved risk modeling, fraud detection, and portfolio optimization are the transformations new financial strategies will undergo.
- Climate Science: Advanced simulations will aid mitigation through powerful and prophetic long-range predictions on the evolution of regional and seasonal climate changes.
- Supply Chain and Logistics: Features of this software-independent technology depend much upon proprietary secrets that aim at increasing variability, hence decreasing constraint, in any model for optimization.
Potential for QNNs to Revolutionize AI
It is likely that QNNs will usher in the advent of the AI Systems:
- More Efficient: Organizations should reduce their energy use without sacrificing performance levels.
- Highly Scalable: Capable of processing vast datasets without bottlenecks.
- Adaptive: The use of quantum properties enables the system to adjust to variable, complex environments in real time.
The evolution of these technologies will transform the AI Microsoft foundation and enhance its operational efficiency as well as its effectiveness.
Conclusion
Quantum neural networks have transcended artificial intelligence. They combined the laws of quantum mechanics with those of the neural network. The output of QNN is associated with solving previously unsolved problems to changing norms in the way industries operate, making it a valuable discovery.
We must use QNNs accurately in all aspects. This will enable us to achieve the remaining benefits that are yet to be unlocked. It will also encourage investment in collaborated efforts among different sectors. If we choose to be responsible in developing QNNs. We will also be paving the way for innovations that can benefit our society.